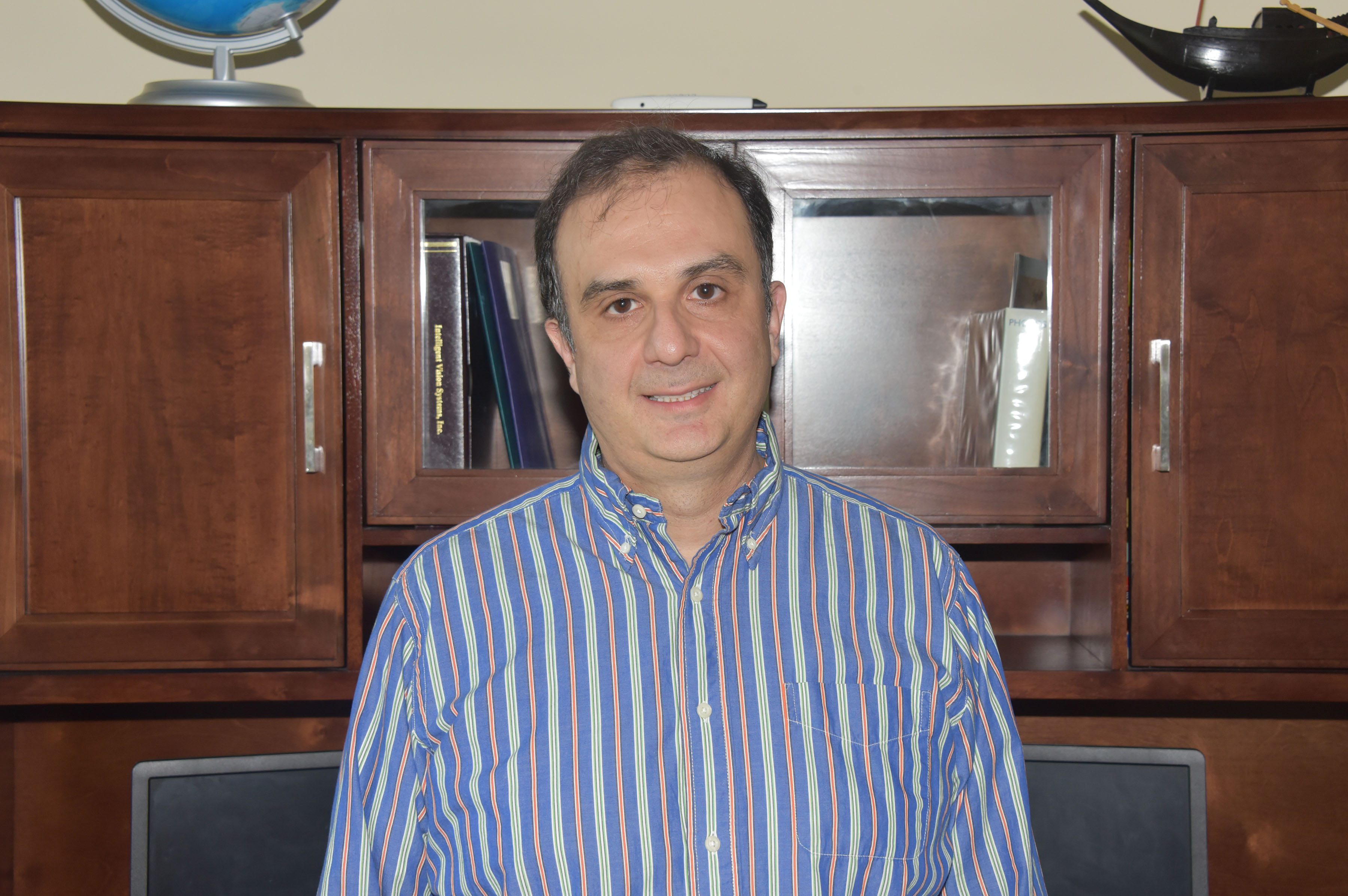
DoD Grant funds cell research automation development
Dr. Sokratis Makrogiannis, a Delaware State University associate professor of mathematics, has been awarded a three-year $435,000 grant from the U.S. Department of Defense’s Army Research Office to develop a novel automated method to study cells.
Dr. Makrogiannis – who teaches and does research within the University’s Division of Physics, Engineering, Mathematics, and Computer Science – will use the funding to develop techniques for fully automating the observation and documentation of cell segmentation, tracking, event handling and quantification.
The funding will support the development of automated methods designed to successfully detect and track cells, and thereby enable the analysis of their static and dynamic behavior – including cell morphology, cell migration and changes in cell states.
Dr. Makrogiannis’ project represents the next stage of innovation in the use of time-lapse microscopy, which has made it possible to observe and quantify cell cycle progression, migration and the growth control of live cells. Imaging and quantitative analysis techniques are being systematically integrated in biological and medical studies that investigate cellular immune response, embryonic development, tumor formation, and drug effects.
He added that the large volume of data produced by microscopy and imaging further emphasizes the need for automated and robust techniques that can address the challenges in accurate detection and segmentation as well as tracking.
“The shortcoming of most methods by biologists and pathologists are when you have a cell segmentation, it take many long hours to observe and document it, and it may result in errors,” Dr. Makrogiannis said. “It will be meaningful to automate the process.”
Dr. Joseph Myers, program manager Army Research Office (an element of the U.S. Army Combat Capabilities Development Command’s Army Research Laboratory), said the new mathematical methods being pursued by this research may help fully automate the process of segmenting visual images of groups of cells and other structures into individually recognized and classified cells, including while in motion or evolving.
“The novelty in stitching together significant aspects between images and not getting fooled by noise, gaps, or bifurcations will be an important advance that is different from current machine learning methods and may hold an advantage over them in certain ambiguous cases,” Dr. Myers said. “If successful, this research will help advance the automation of a whole lane of tasks for cell/microbiology research.”